Designing Artificial Intelligence Software to Improve Student Learning
by Tom Hanlon / Mar 8, 2023
Can artificial intelligence be used to improve students’ learning—while keeping their data private and being fair and effective for students from all demographic backgrounds? Nigel Bosch and Dong Wang aim to find out.
Have you ever gone into a test pretty confident you were going to ace it, only to come out with a C+? If so, you’re not alone.
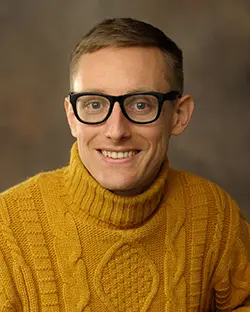
“From a number of studies, the overall pattern is that almost everybody overestimates their knowledge,” says Nigel Bosch, assistant professor in the School of Information Sciences (iSchool) with a joint appointment in the Educational Psychology Department at the College of Education.
That overestimation, of course, can lead to shock, dismay, and lower-than-expected grades.
Three-year AI Study
Bosch and Dong Wang, associate professor in the iSchool, have undertaken a three-year study, funded by the National Science Foundation under its Research on Emerging Technologies for Teaching and Learning program, to help students more accurately estimate their knowledge by using Artificial Intelligence (AI). Their study is titled “A Metacognitive Calibration Intervention Powered by Fair and Private Machine Learning.”
“As you learn more, you learn that there’s always more to learn, and you realize how much you don’t know,” says Bosch. “There’s a lot of research in educational psychology that shows how important it is to be able to estimate how much you know and what you know so you can more effectively plan and study the right things and with the right amount of time.”
Getting Feedback Before it's Too Late
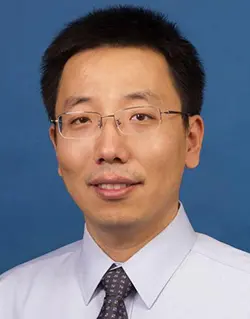
“Our project is about designing some AI software that can give students feedback before it’s too late for them to make changes in their study behaviors,” says Wang.
While the project is still in its early stages, it’s possible that the software can provide specific suggestions for study focus, Wang adds.
“The simplest version of feedback is just ‘You thought you were going to get an 85 on the midterm but we think you’re going to get a 75,’” Bosch explains. “But that’s not very specific. We’re thinking about how we could break that down by topic: Like, here’s how well you think you know this particular topic, and here’s how well the AI method thinks you know it.”
From there, Bosch says, the students could be given reflection exercises, such as summarizing a topic and then seeing how that summary stacks up to what the domain expert thinks about the topic.
“We’re trying to approach the feedback from multiple angles but also giving the students more active learning opportunities to teach them how to improve their metacognitive calibration,” Bosch says.
Multiple Studies
Their study is in the early stages, but Bosch says they are planning to do two major studies, the first with about 200 students in a controlled environment. “In year three of the project, we’ll take everything we’ve learned and try it out in a large undergraduate statistics class that usually has about thirteen hundred to fifteen hundred students,” he says. “We’re hoping to get about five hundred students to participate and try out what we have in a very real-world kind of environment.”
Privacy and Fairness Issues
Two critical factors will be front and center throughout the studies: protecting students’ privacy and addressing fairness issues. The iSchool has recently developed a machine-learning model that tackles both issues.
“We don’t want students’ data to be shared with anybody, and we definitely care about fairness because students are from different demographic groups,” Wang explains. The model needs to not be biased toward a majority population group because that could result in poor performance for minority student populations, he says.
In working through the fairness issue, Wang cautions that there is a tradeoff. “If you do all this for fairness, then you might lose performance,” he says. “Your accuracy could be low across all groups. So, we have to play that tradeoff between performance and fairness, and also consider the privacy issue.”
To maintain privacy, students’ raw data—for example, test scores—are not collected. These data are on the students’ own computers or tablets. Data abstracted by the AI model doesn’t recover that raw data.
Hoped-for Outcomes
The researchers are hoping for several good outcomes.
“Hopefully, we’ll see improved learning for students that use the software that we ultimately design to improve their ability to estimate their knowledge,” Bosch says. “That’s an applied practical outcome. There are also some theoretical outcomes, some technical methods that need to be explored and developed to be able to achieve these privacy and fairness goals, as well as theoretical educational psychology questions that need to be answered or explored surrounding how we actually improve students’ metacognitive calibration. There are many possible interventions that we could provide the students—tips or feedback with very open-ended space that we need to explore.”
Bosch also hopes to learn the mechanism that improves the learning. “If students are better able to estimate how well they do, what effect does that have on their behavior, for example,” he says. “Hopefully, they will acquire some new skills they can apply not just in the courses or topics that we will study in this project, but across their courses.”
Another desired outcome, Wang adds, is to showcase that AI can be good, fair, and trustworthy under some educational circumstances.
The Bottom Line: Helping Students Learn
The bottom line, of course, is helping students learn what they need to learn.
“Generally, the higher-performing students are more or less accurate in estimating their knowledge,” Bosch says. “They may even underestimate it at times.”
Wang agrees. “The primary focus is on those who overestimate their performance. We want to help them improve their performance before it’s too late.
“But there is a small group of students who underestimate their performance. They spend way more time than necessary, and they get an A+, but they can make better use of their time. If our software can help them to better manage their time, why not?”